Hi, everyone! I am Paschal Alaemezie, a Gold Microsoft Learn Student Ambassador. I am a student at the Federal University of Technology, Owerri (FUTO). I am interested in Artificial Intelligence, Software Engineering, and Emerging technologies, and how to apply the knowledge from these technologies in writing and building cool solutions to the challenges we face. Feel free to connect with me on LinkedIn and GitHub or follow me on X (Twitter).
In my previous article, I wrote about Recommendation Engines and gave a walkthrough on how to train a simple recommendation engine using the Azure Machine Learning Designer via the Azure portal. In this article, I will give a walkthrough on how to replicate this training using Azure Machine Learning Designer via the new Azure AI Studio.
The new Azure AI Studio is a comprehensive platform designed to facilitate the development, management, and deployment of AI applications. It offers a user-friendly interface with drag-and-drop capabilities for model creation, alongside advanced features for model management and scalability. The platform supports automated machine learning to optimize model selection and tuning. It is suitable for creating custom AI solutions, including chatbots and other AI-driven applications, with a focus on collaboration, efficiency, and responsible AI practices. Azure AI Studio is available in public preview, providing a glimpse into the future of AI development tools.
An Azure subscription is required to carry out the activities in this article. If you are a student, you can use your university or school email to sign up for a free Azure for Students account and start building on the Azure cloud with a free $100 Azure credit.
Activity 1: Create a New Training Pipeline
Step 1: Setting up your Azure AI Studio workspace
- Open your web browser and go to ai.azure.com to open the new Azure AI Studio
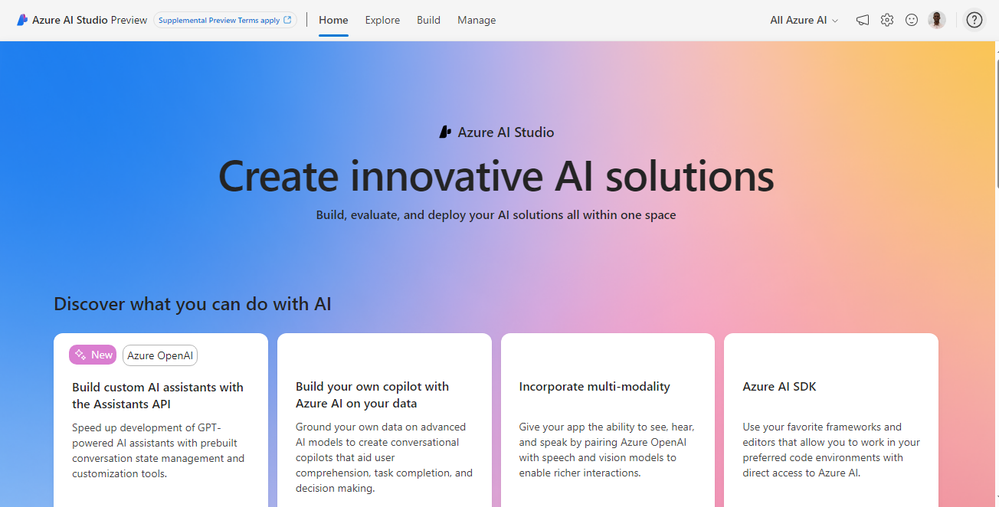
- Go to Build on the Azure AI Studio and click on it to open the Build environment. Then click on + New project button to open the Create a project environment.
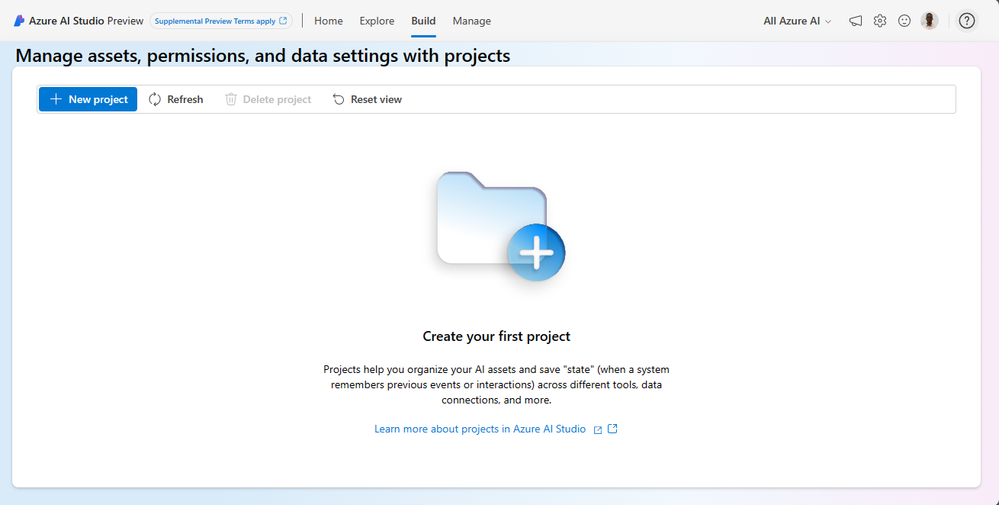
Step 2: Creating your project
- For the Project details section:
- At Hub name, key in your preferred name for your project’s hub in the input box provided.
- At Subscription, select your existing subscription from the drop-down menu.
- Select your Resource group. If you have any existing resource group, select it from the drop-down menu. Otherwise, click on Create new to create a new resource group, and click OK after that.
- At Location, select your location from the drop-down menu. Then, click on the Next button at the bottom of the screen to go to the Review and finish.
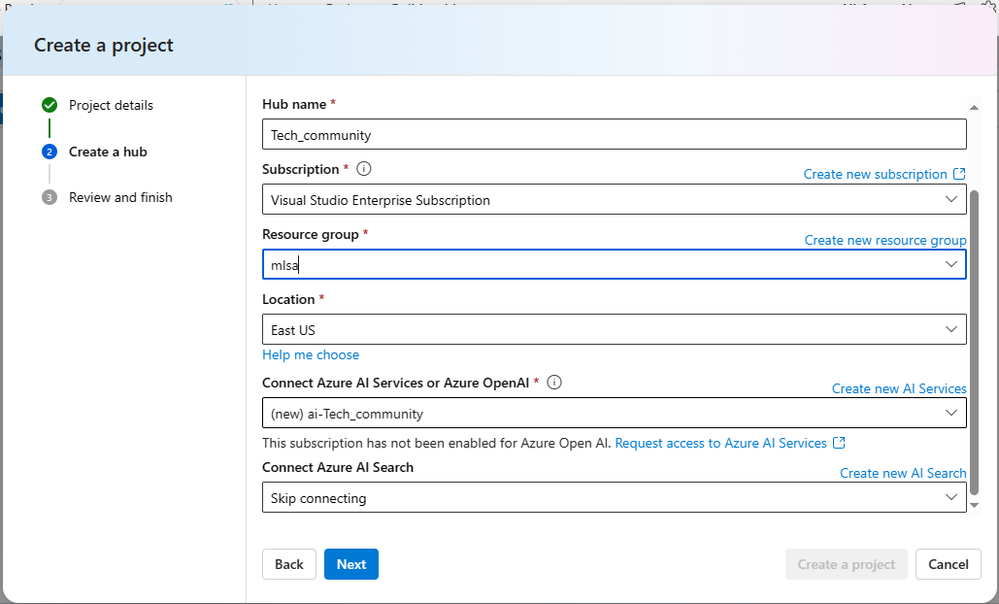
- At the Review and finish section, click on Create a project button at the bottom of the screen to provision your workspace on Azure AI Studio.
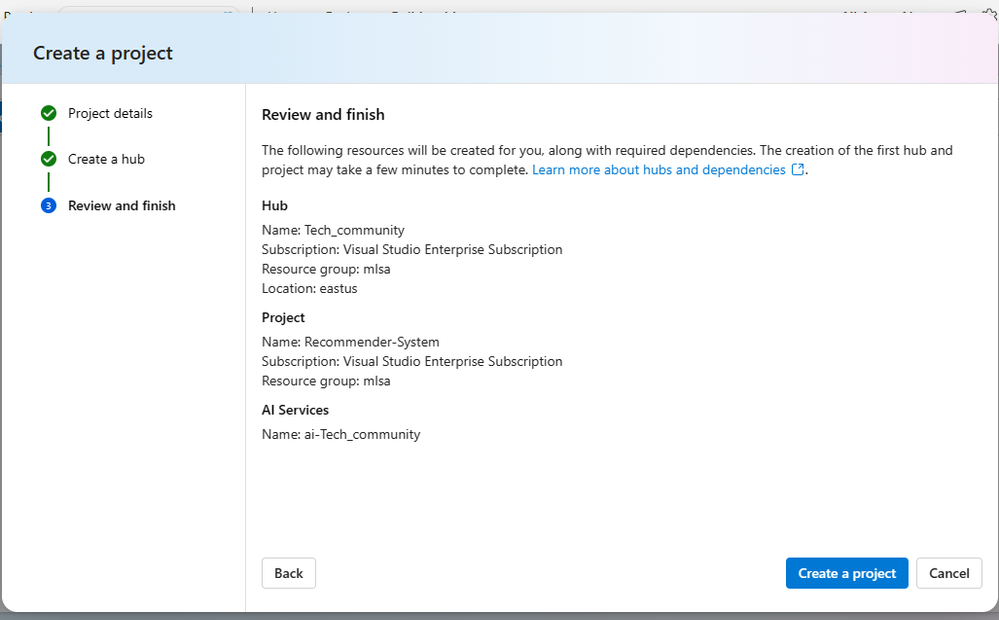
- Your provisioned workspace will display the window below. Go to the All Azure AI at the upper right of the screen and select the Azure Machine Learning Studio from the drop-down menu.
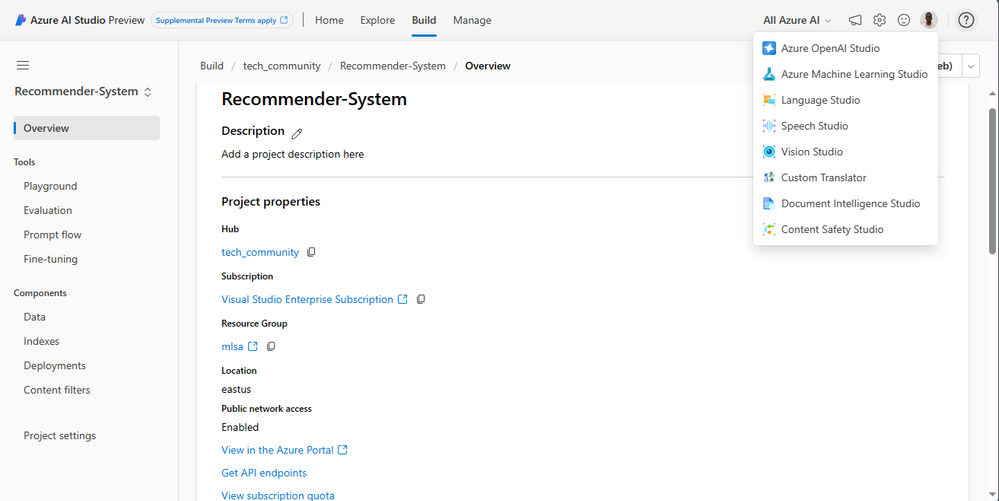
- In the Azure Machine Learning studio, select Designer from the navigation pane on the left-hand side. This will open the Designer environment where you can select a new pipeline if there is no existing pipeline.
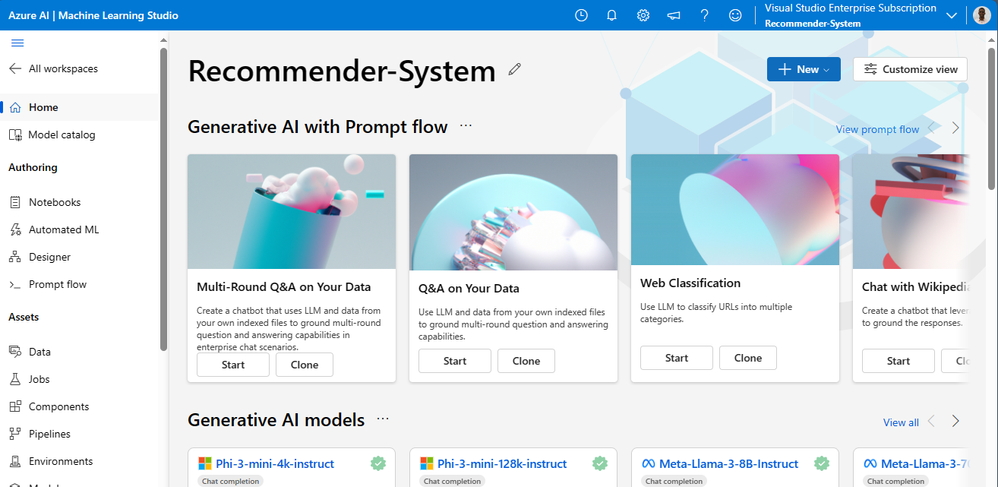
- In the Designer environment, select the Classic prebuilt component. Then click on the Create a new pipeline using classic prebuilt components. This will open a visual pipeline authoring editor.
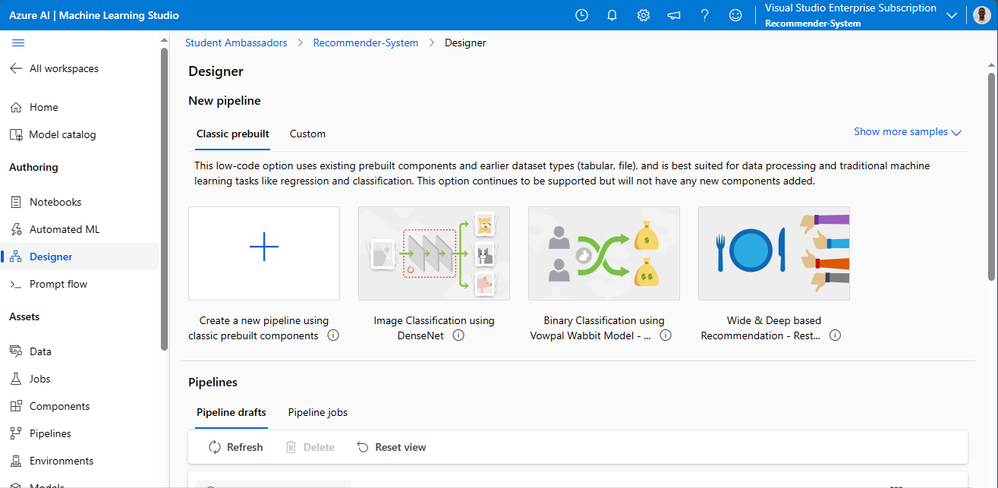
Step 3: Add Sample Datasets
- In the left navigation pane of the Authoring editor, click the Asset library and go to the Component section. Under Component, click on Sample data.
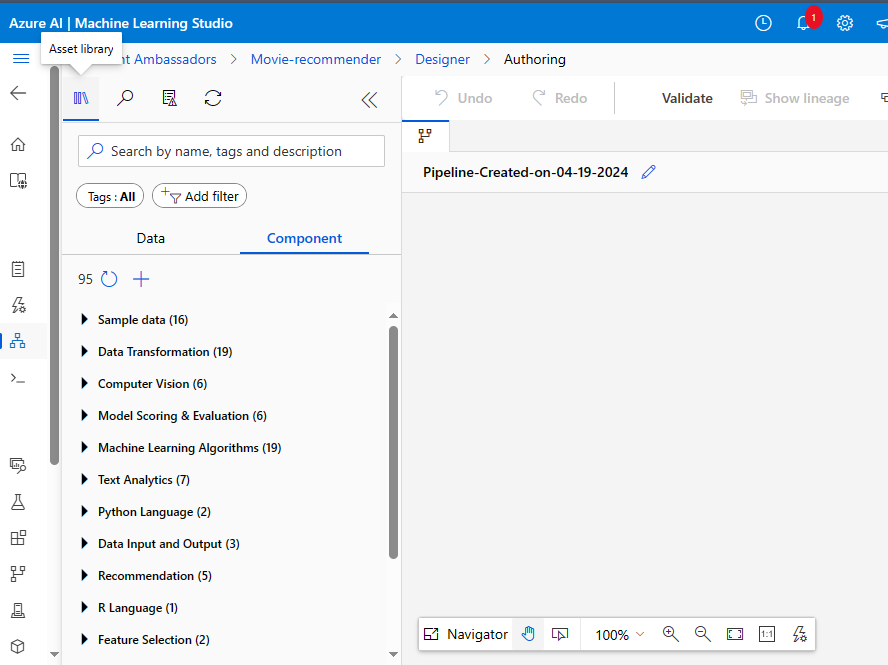
- In the Sample data, scroll down to the Movie Ratings, and IMDB Movie Titles. Drag and drop the selected datasets onto the canvas.
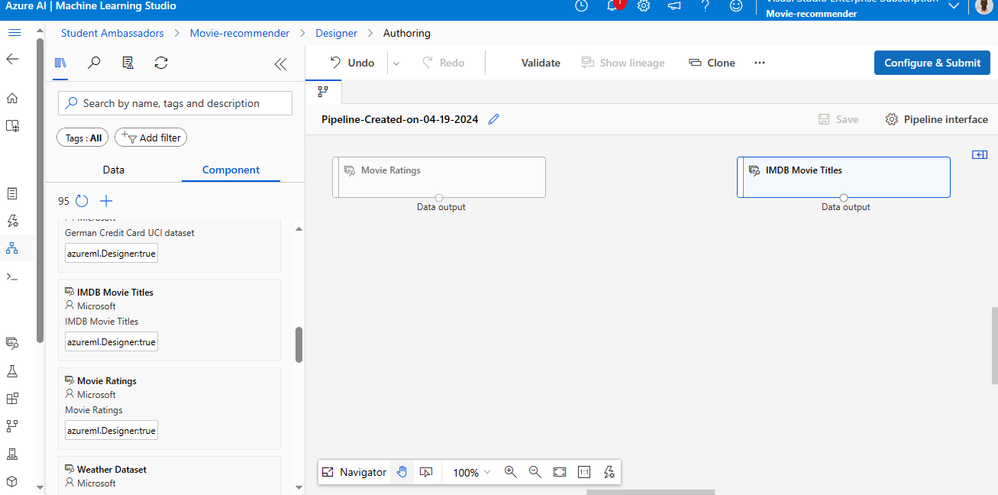
Step 4: Join the two datasets on Movie ID
- Close the Sample data drop-down menu. From the Data Transformation section in the left navigation, select the Join Data prebuilt module, and drag and drop the selected module onto the canvas
- Connect the output of the Movie Ratings module to the first input of the Join Data module.
- Connect the output of the IMDB Movie Titles module to the second input of the Join Data module.
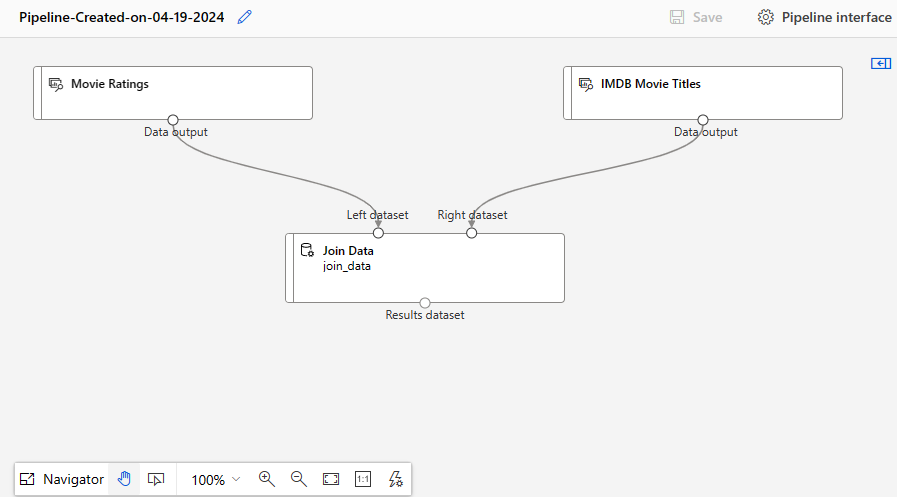
- Select the Join Data module. Click the navigation button at the upper right of the canvas to open the Join Data module window.
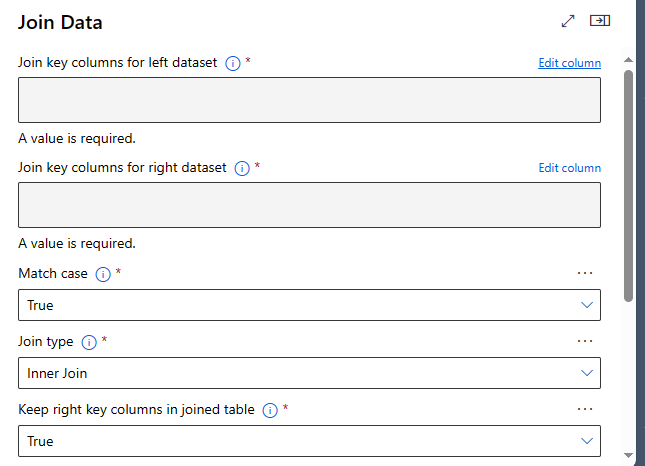
- Select the Edit column link to open the Join key columns for the left dataset editor. Select the MovieId column in the Enter column name field and click Save.
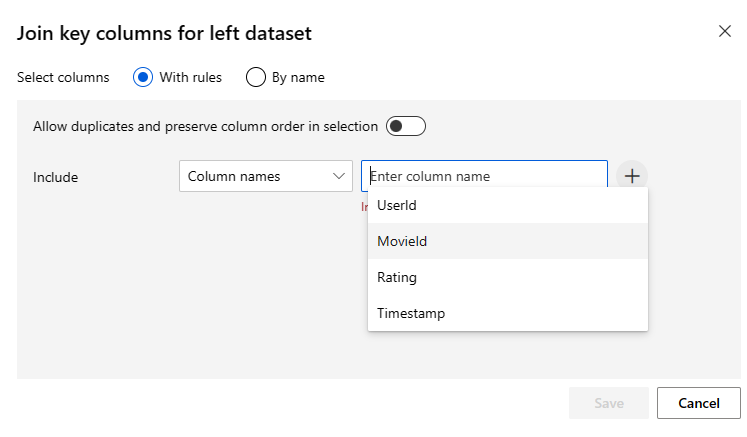
- Select the Edit column link to open the Join key columns for the right dataset editor. Select the Movie ID column in the Enter column name field and click Save. Then, close the Join Data window.
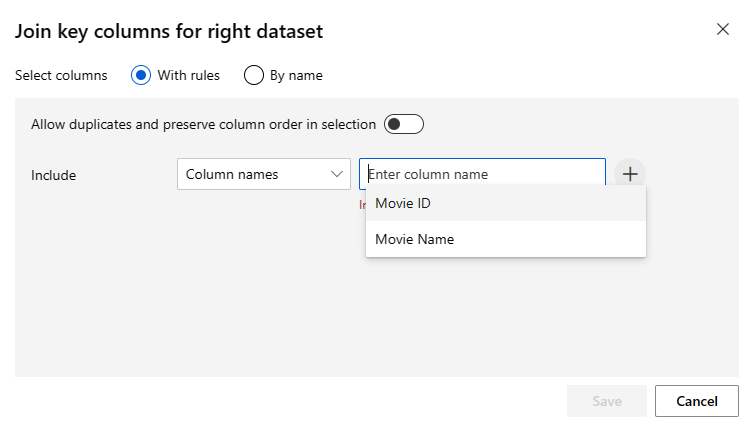
Step 5: Select Columns UserId, Movie Name, and Rating using a Python script
- From the Python Language section in the left navigation, select the Execute Python Script prebuilt module. Drag and drop the selected module onto the canvas. Then, connect the Join Data output to the input of the Execute Python Script module.
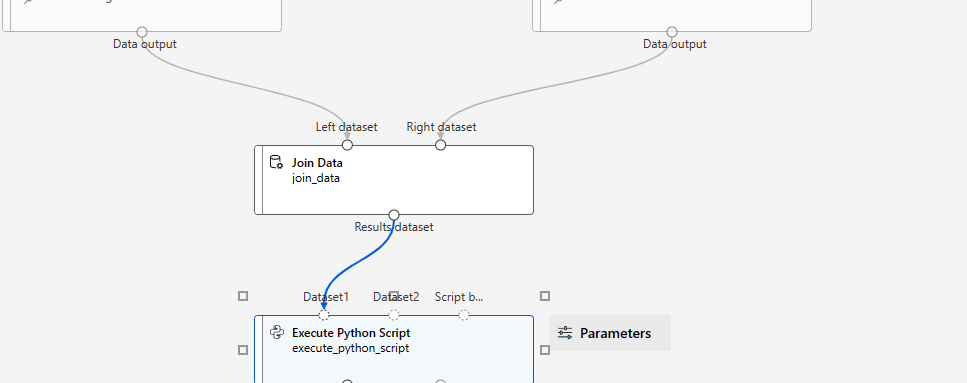
- Select Edit code to open the Python script editor, clear the existing code and then enter the following lines of code to select the UserId, Movie Name, and Rating columns from the joined dataset. Ensure best practice by indenting only the second and third lines of your code.
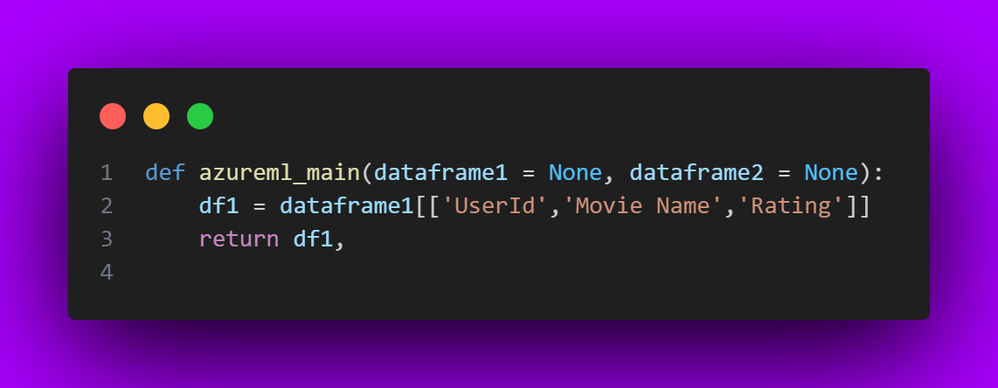
Step 6: Remove duplicate rows with the same Movie Name and UserId
- From the Data Transformation section in the left navigation pane, select the Remove Duplicate Rows prebuilt module from the drop-down menu, and drag and drop the selected module onto the canvas.
- Connect the first output of the Execute Python Script to the input of the Remove Duplicate Rows module.
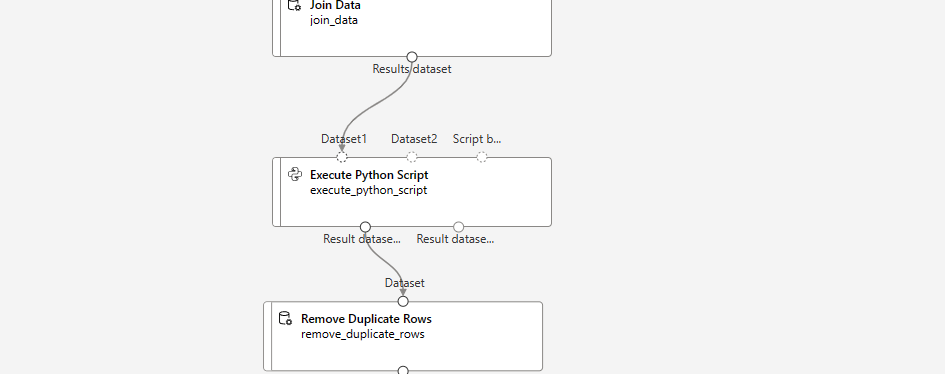
- Select the Edit column link to open the Select column editor. Click the navigation button at the upper right of the canvas to open the Remove Duplicate Rows module window.
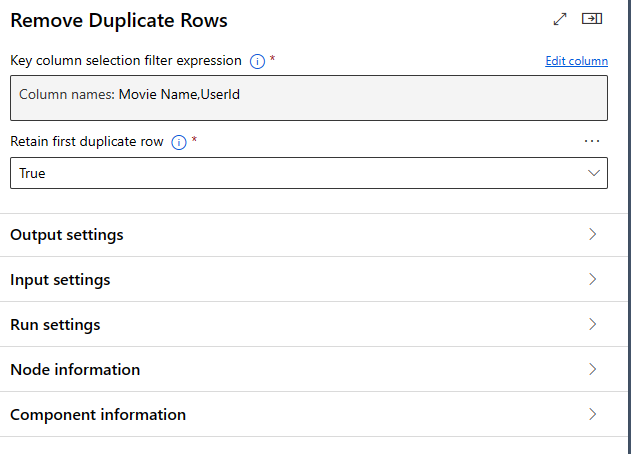
- Enter the following list of columns to be included in the output dataset: Movie Name, UserId. Then, click Save.
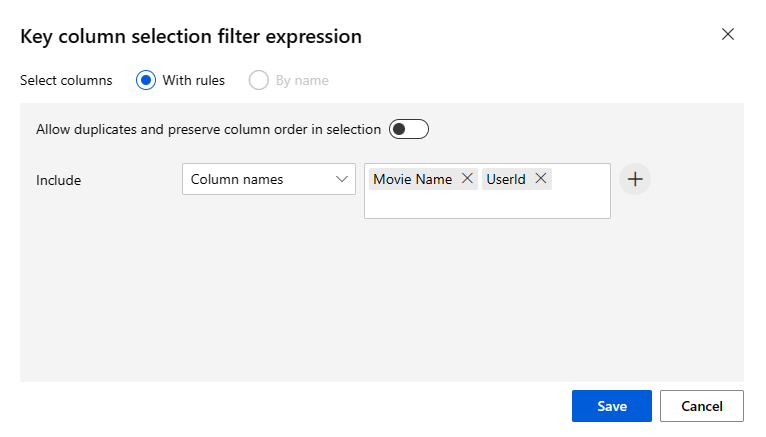
Step 7: Split the dataset into a training set (0.5) and a test set (0.5)
- From the Data Transformation section in the left navigation select the Split Data prebuilt module and drag and drop the selected module onto the canvas, then connect the Dataset to the Split Data module.
- Click the navigation button at the upper right of the canvas to open the Split Data module window. Ensure that the Fraction of rows in the first output dataset: 0.5
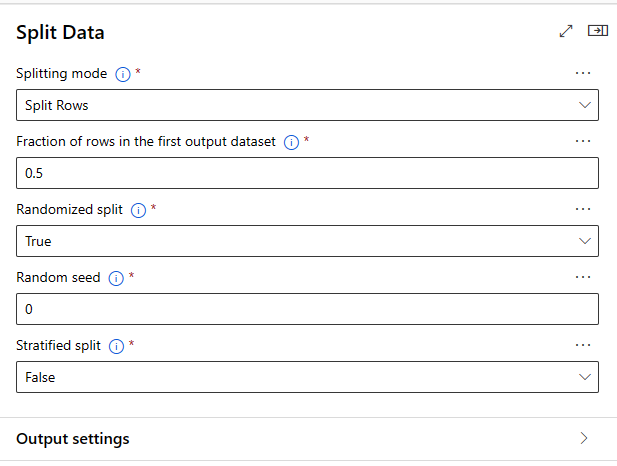
Step 8: Initialize Recommendation Module
- From the Recommendation section in the left navigation pane, select the Train SVD Recommender prebuilt module and drag and drop the selected module onto the canvas. Then, connect the first output of the Split Data module to the input of the Train SVD Recommender module.
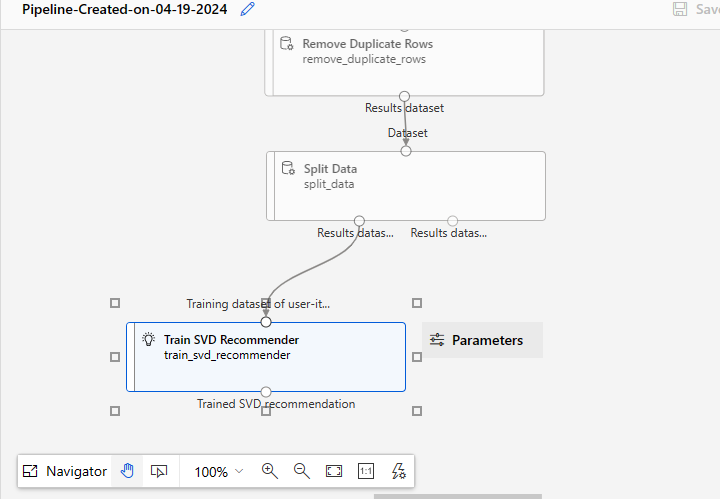
- Click the navigation button at the upper right of the canvas to open the Train SVD Recommender module window. Set Number of factors: 200. This option specifies the number of factors to use with the recommender.
- Number of recommendation algorithm iterations: 30. This number indicates how many times the algorithm should process the input data. The default value is 30.
- For Learning rate: 0.001. The learning rate defines the step size for learning.
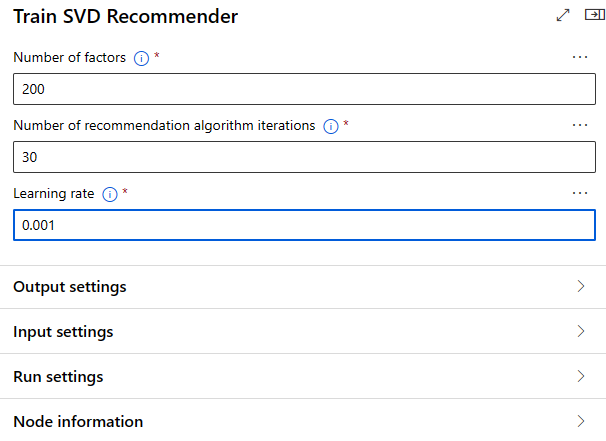
Step 9: Select Columns UserId, Movie Name from the test set
- From the Data Transformation section in the left navigation pane, select the Select Columns in Dataset prebuilt module and drag and drop the selected module onto the canvas. Then, connect the Split Data second output to the input of the Select columns in Dataset module.
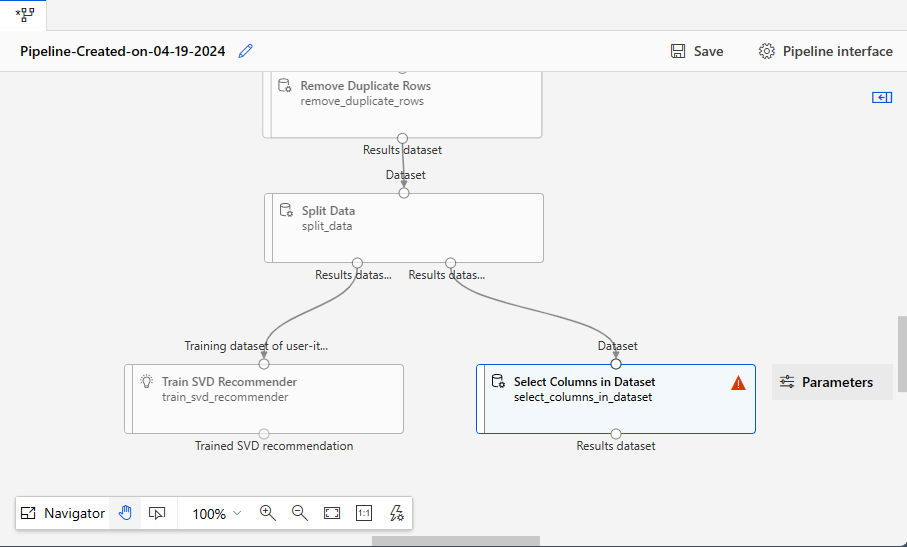
- Click the navigation button at the upper right of the canvas to open the Select Columns in Dataset module window. Select the Edit column link to open the Select columns editor.
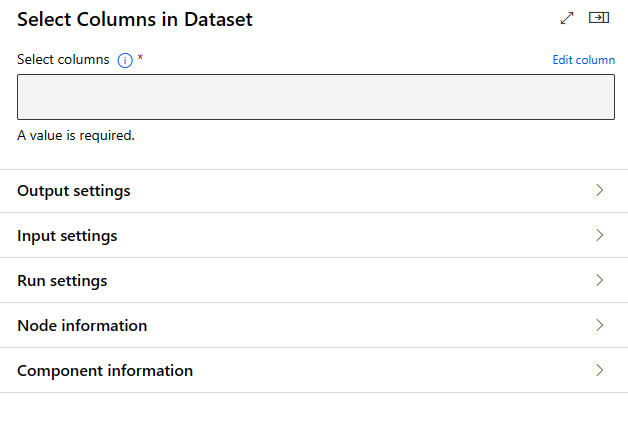
- Enter the following list of columns to be included in the output dataset: UserId, Movie Name and Click Save.
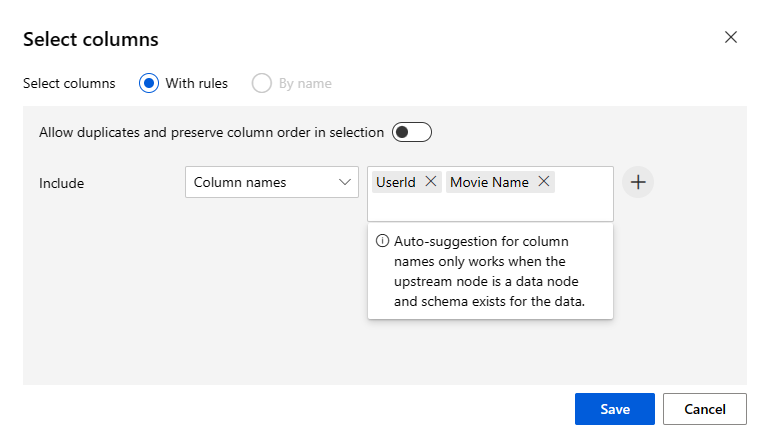
Step 10: Configure the Score SVD Recommender
- From the Recommendation section in the left navigation pane, select the Score SVD Recommender prebuilt module and drag and drop the selected module onto the canvas
- Connect the output of the Train SVD Recommender module to the first input of the Score SVD Recommender module, which is the Trained SVD recommendation input.
- Connect the output of the Select Columns in Dataset module to the second input of the Score SVD Recommender module, which is the Dataset to score input.
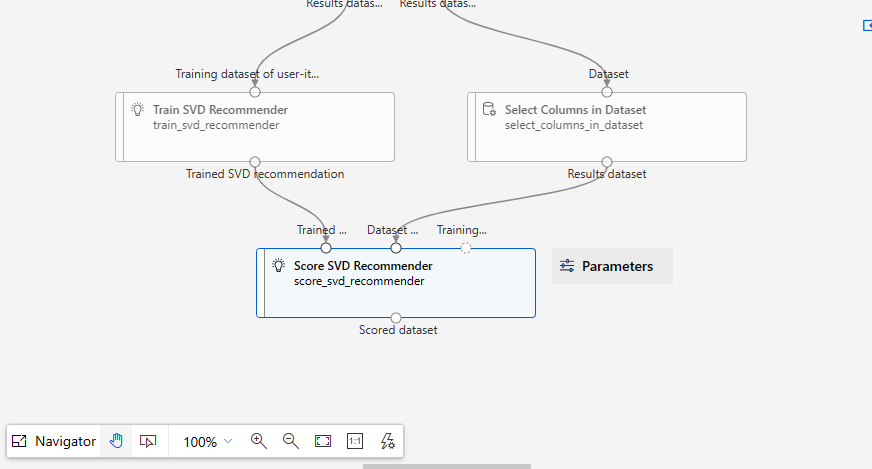
- Open the Score SVD Recommender module on the canvas by clicking on the navigation button at the upper right of the canvas. Set the Recommender prediction kind: Rating Prediction. For this option, no other parameters are required.
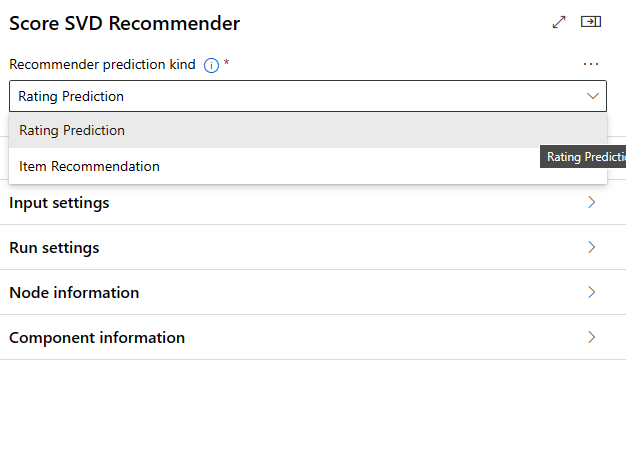
Step 11: Setup Evaluate Recommender Module
- From the Recommendation section in the left navigation pane, select the Evaluate Recommender prebuilt module and drag and drop the selected module onto the canvas.
- Connect the Score SVD Recommender module to the second input of the Evaluate Recommender module, which is the Scored dataset input.
- Connect the second output of the Split Data module (train set) to the first input of the Evaluate Recommender module, which is the Test dataset input.
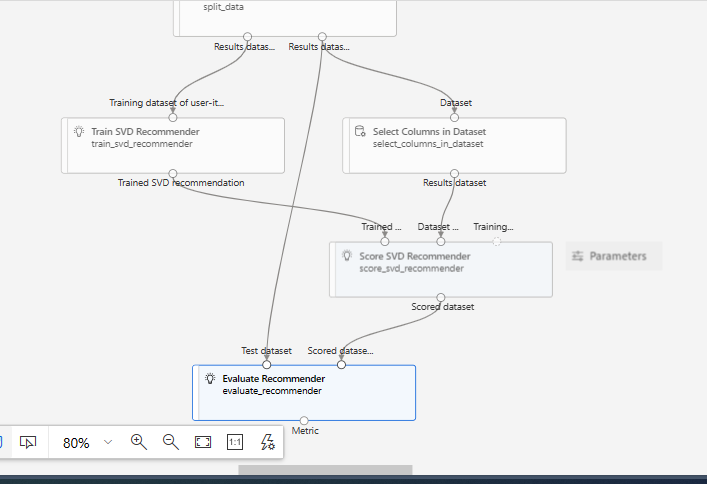
Activity 2: Submit Training Pipeline
- In the Authoring editor, ensure that you have AutoSave enabled. Then click on Configure & Submit at the upper right-hand side of your screen.
- For the Set up pipeline job window: In the Basics section, click the Create new button under the Experiment name. Type your new experiment name and click the Next button at the bottom of the screen.
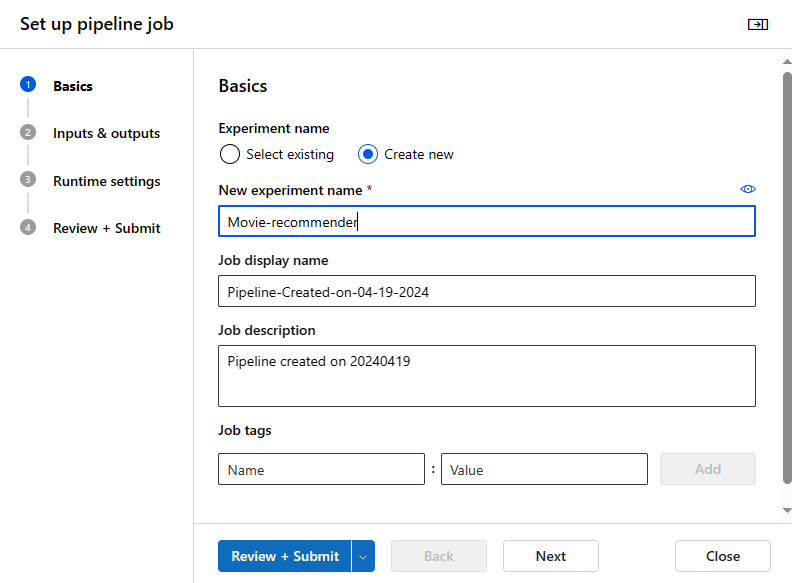
- In the Inputs & outputs section, click the Next button at the bottom of the screen.
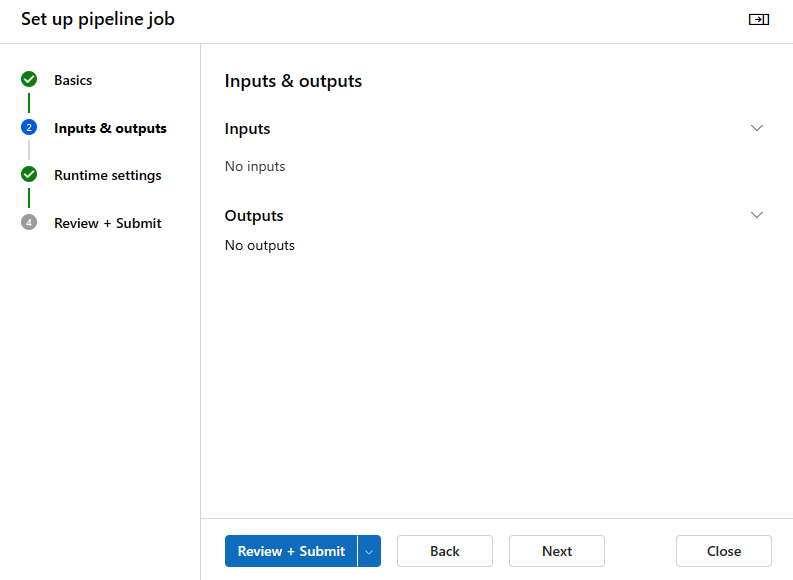
- In the Runtime settings section: skip the Default compute. Go to the select compute type and select Compute instance from the drop-down menu. Under the Select Azure ML compute instance, click on Create Azure ML compute instance. The Create compute instance will open in another environment.
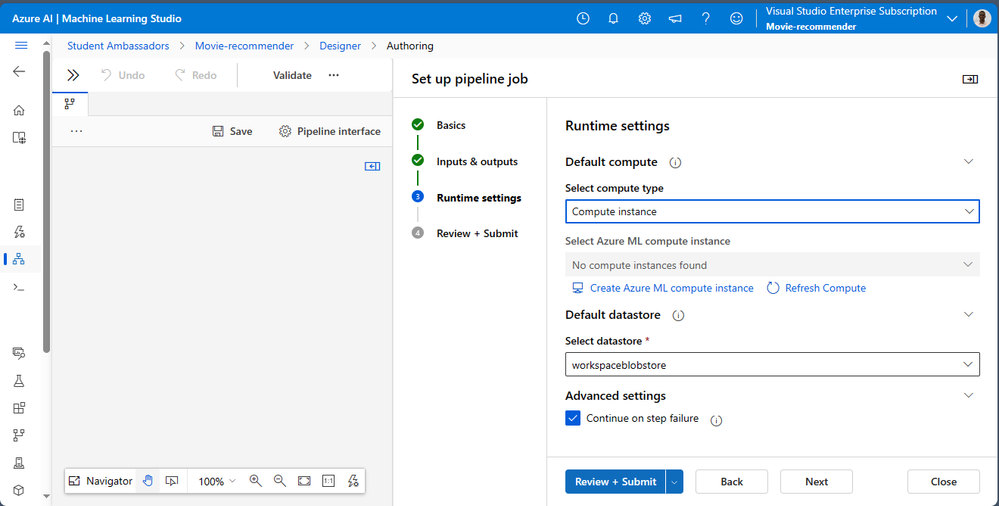
- In the Create compute instance window, type in your compute name under the Compute name tab. Then, select the CPU button under the Virtual machine type.
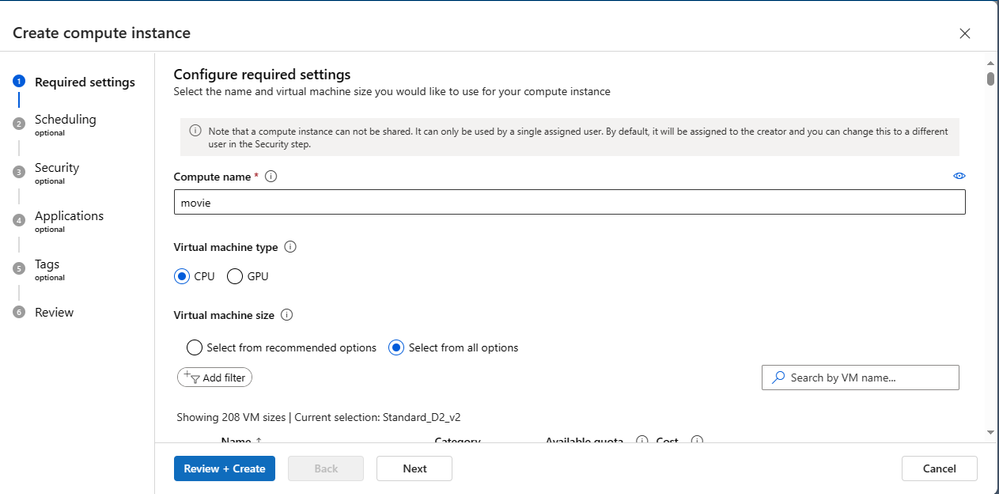
- While authoring this article, I had to select my virtual machine first to enable the Compute name tab. You may or may not encounter this issue. I selected the Standard_D2_v2 virtual machine for this training. After that, click the Review + Create button at the end of the screen, to take you back to the Runtime settings window.
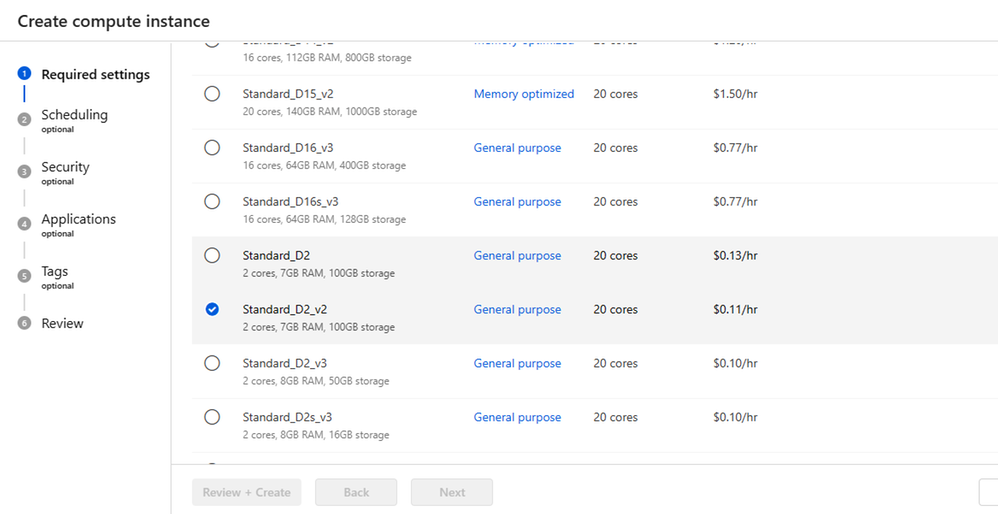
- Back to the Runtime settings window. At the Select Azure ML compute instance, Select the compute instance that you have created. Here, I selected the movie instance from the drop-down menu. Note that your newly created compute instance will take some time to be provisioned and appear in your drop-down menu. Go to the Advanced settings and ensure that the Continue on step failure box is checked. Then, click the Review + Submit button at the end of the screen.
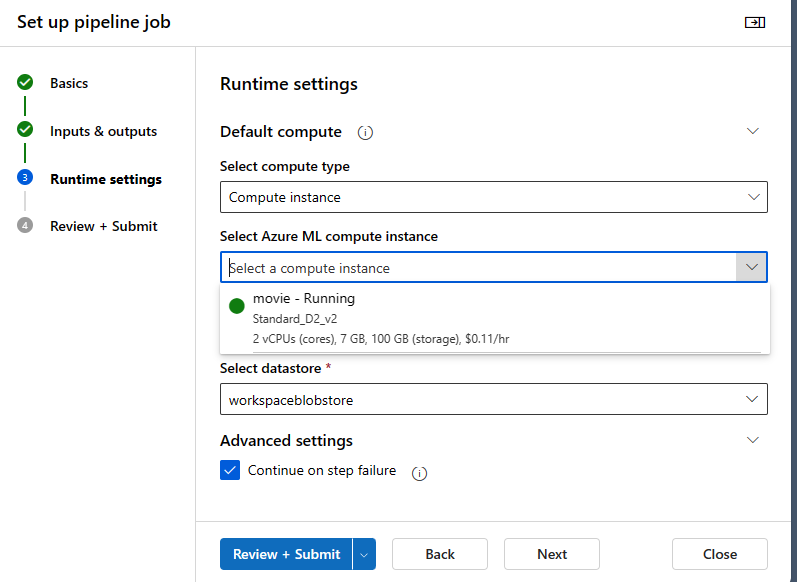
- At the Review + Submit section, ensure that your provided details are correct. Then, click the Submit button at the end of the screen.
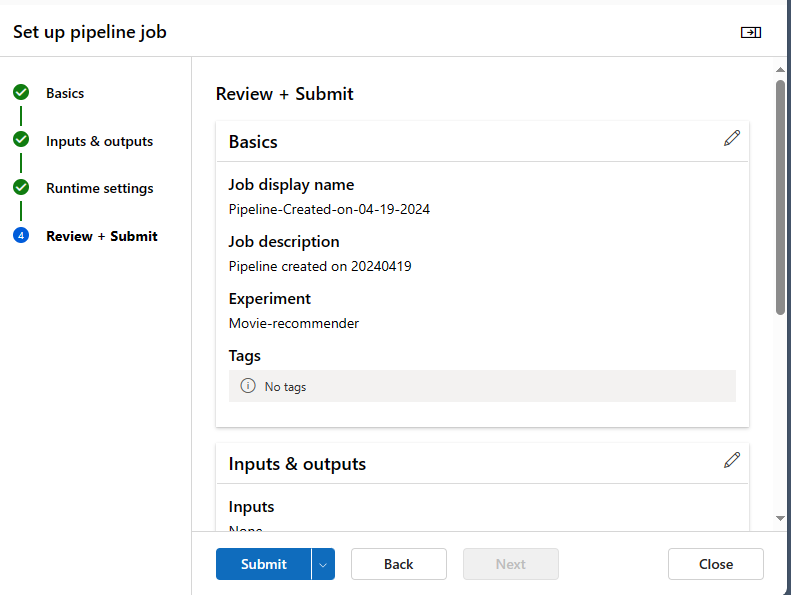
Activity 3: Visualize Scoring Results
Step 1: When your pipeline is submitted and your model training is completed, at the left navigation pane, go to Jobs under Asset and click on the name of your completed pipeline.
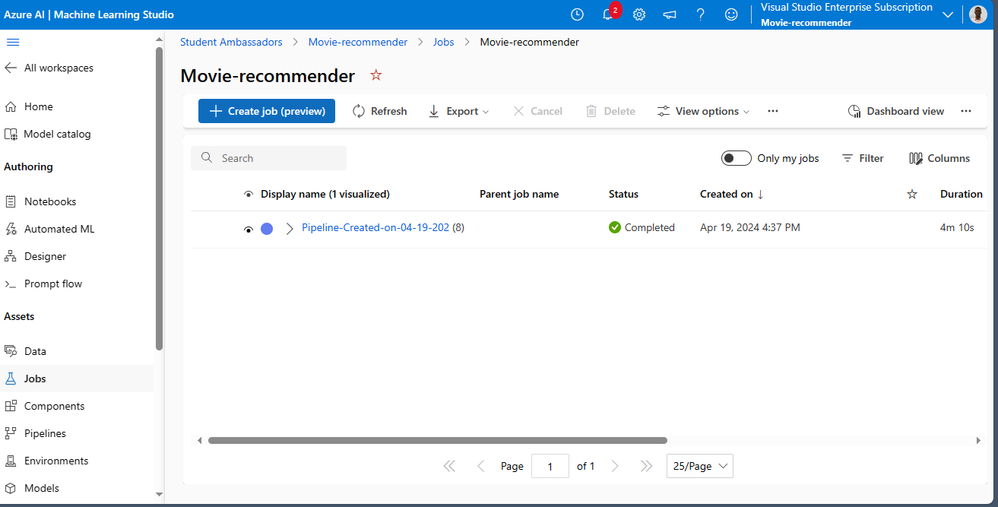
Step 2: Visualize the Scored dataset
- Go to the Score SVD Recommender module on the canvas and right-click on it. Select Preview data and click on Scored dataset.
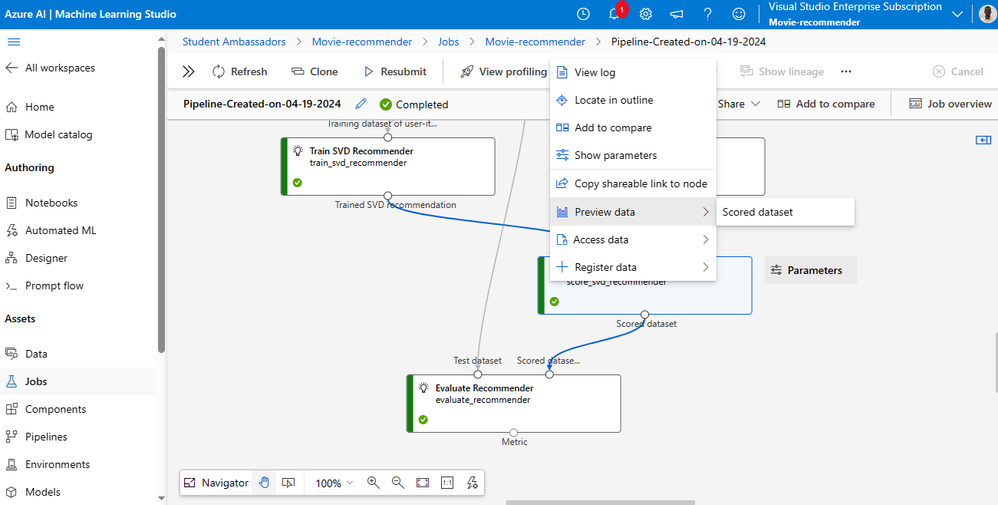
- Observe the predicted values under the column Rating.
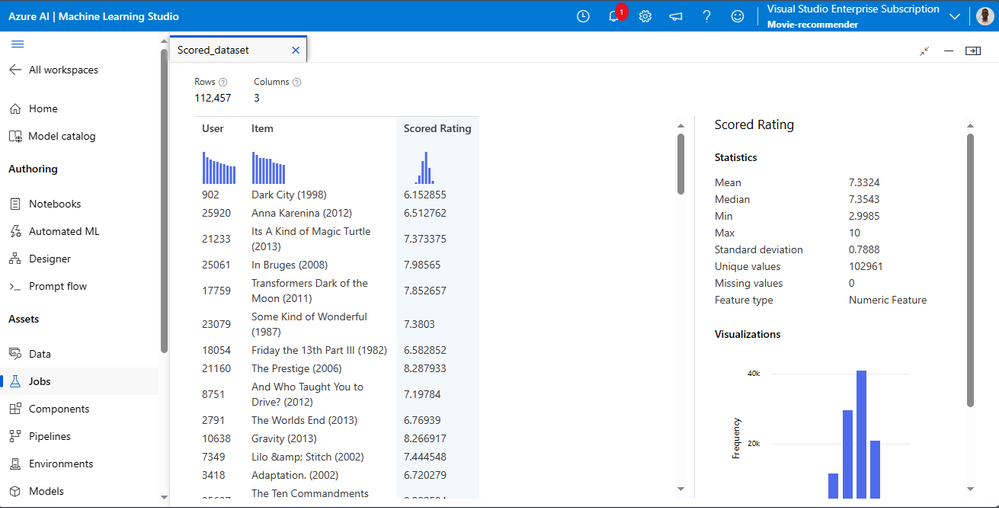
Step 3: Visualize the Evaluation Results
- Go to the Evaluate Recommender module on the canvas and right-click on it. Select Preview data and click on Metric.
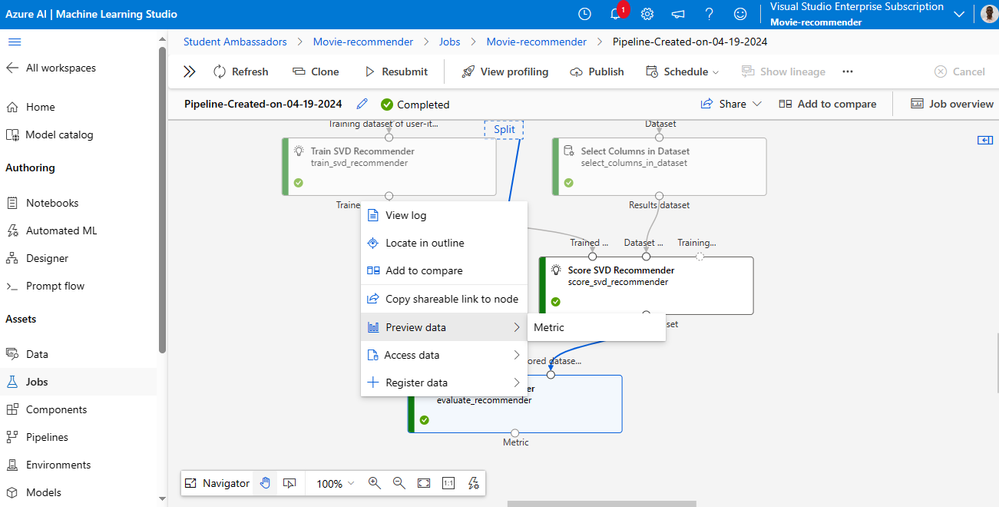
- Evaluate the model performance by reviewing the various evaluation metrics, such as Mean Absolute Error, Root Mean Squared Error, etc.
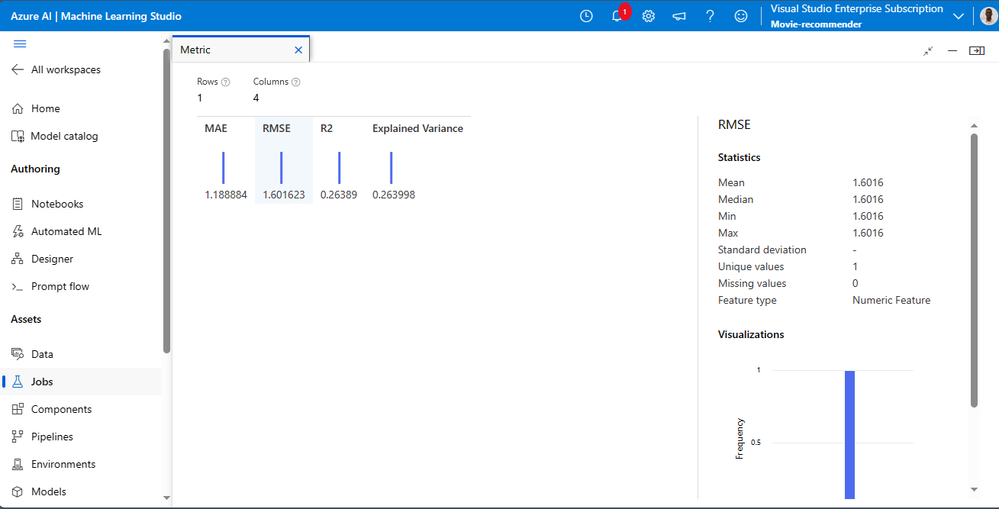
Next step
Congratulations, on making it this far. Stay tuned for my next blog on the amazing solutions you can build using the Azure AI Studio.
For enthusiasts and professionals alike, you can leverage these resources to stay informed and inspired as you embark on your AI journey: